Resources
and Environmental Scientific Dataset of the Mongolian Plateau
Wang, J. L.1,2* Li, K.1,2 Altansukh, O.3 Xu, S. X.1,2 Wei, H. S.4
1. State
Key Laboratory of Resources and Environmental Information System, Institute of
Geographic Sciences and Natural Resources Research, Chinese Academy of
Sciences, Beijing 100101, China;
2. College of Resources and Environment, University
of Chinese Academy of Sciences, Beijing 100049, China;
3.
Environmental Engineering Laboratory, Department of Environment and Forest
Engineering, School of Engineering and Technology, National University of
Mongolia, Ulaanbaatar 14201, Mongolia;
4. School of Geography, Beijing Normal University,
Beijing 100875, China;
Abstract: The
Mongolian Plateau is a key region for the green development of the
China-Mongolia-Russia Economic Corridor,
which is of great significance for the ecological security of Asia. In this
study, a series of scientific data products on the resources and environment of
the Mongolian Plateau were developed using remote sensing and geographic information
system (GIS) technologies to address the resource and ecological problems faced
by the region, such as land degradation, water scarcity, and frequent sand and
dust storms. The dataset includes sub-datasets of land cover, spring sandstorm
distribution, grass production estimation, and surface water distribution, with
the aim of providing scientific support for the sustainable development of the
region. These results effectively reflect the ecological and environmental
problems of Mongolia and the Mongolian Plateau. The developed data products
meet high standards of accuracy and reliability and can effectively support the
monitoring and management of ecological barriers in the Mongolian Plateau.
Keywords: Mongolian plateau; land cover; dust storms; grass yield; surface water
DOI: https://doi.org/10.3974/geodp.2024.04.11
CSTR: https://cstr.escience.org.cn/CSTR:20146.14.2024.04.11
1 Introduction
The
Mongolian Plateau is a key region for the green development of the ??Belt and
Road?? China-Mongolia-Russia Economic Corridor. It lies in the transition region
between the Siberian taiga and the Asian desert steppe and plays an important
role as an ecological barrier in northern China. This region is not only
crucial to the prosperity of the Chinese nation but also has a significant
impact on Asian civilization and geopolitical patterns. However, it faces
severe ecological and environmental challenges such as land degradation,
desertification, frequent sandstorms and dust storms, uncontrolled expansion of
animal husbandry, and water scarcity due to global warming and human activities[1]. Mongolia is a global hotspot for desertification research, with
more than 75% of its land area experiencing desertification of varying degrees.
This phenomenon spreads eastward to the high-quality steppe areas such as
Eastern Province and Kent Province, which considerably hinders the realization
of the United Nations Sustainable Development Goal SDG15.3.1 Zero Growth of
Land Degradation. China and Mongolia have been hit by the strongest sandstorms
in the last decade, which have caused great losses to the lives and property of
local people.
Chairman Xi Jinping
visited Inner Mongolia several times in recent years, emphasizing the
importance of building an important ecological security barrier in northern
China. At the 2022 Shanghai Cooperation Summit, the heads of China, Mongolia,
and Russia vowed to extend the construction of the China-Mongolia-Russia
Economic Corridor Development Plan for another 5 years. During a visit by the
Prime Minister of Mongolia to China in 2023, cooperation between China and
Mongolia regarding transboundary ecological management was further
strengthened. However, for historical reasons, the Mongolian Plateau has long
been underappreciated and lacks an accumulation of highly spatially and
temporally resolved scientific data. To cope with the rapid development of Earth
observation and processing technologies, there is an urgent need to develop and
produce refined scientific data regarding the resources and environment of the
Mongolian Plateau.
Oriented to the
paradigm change in geoscience research driven by big data, this study considers
the demand for intelligent computing of ecological barriers on the Mongolian
Plateau as traction, bridging the chain between the data-model-product-scenario
and solving the key data product inversion algorithms, multi-source data
fusion, and knowledge discovery scenarios to support intelligent computing of
large-area geographic units. We will implement the inversion algorithm of
high-resolution surface feature parameter data products on the Mongolian
Plateau and the research and development method of products related to the
ecological security of the Mongolian Plateau to improve the guaranteed ability
of China??s independent data products and maximize their supportive role in the
construction of an ecological barrier on the Mongolian Plateau.
2 Data Development Methods
Targeting
the arid and semi-arid characteristics of the ecological barrier on the
Mongolian Plateau, key indicators were screened in terms of vegetation, soil,
water conditions, and the ecological environment, such as land cover, surface
water, vegetation cover, leaf area index, surface temperature, grassland
biomass, vegetation water supply index, and soil moisture. Analysis and design
of the consistency and standardization of source data indicators for feature
parameter calculation will be conducted. Synergistic technical solutions for
sharing, accuracy validation, and quality evaluation of data products for
intelligent calculation of key feature parameters will be designed to construct
an accurate and shareable system of key surface parameter data products for the
Mongolian Plateau (Figure 1).
The characteristics of the
existing monitoring algorithms for each key parameter were compared and
analyzed based on remote sensing and GIS spatial analysis algorithms. A land
cover production and change monitoring model based on an image segmentation algorithm
was constructed, and the surface temperature inversion split-window algorithm
was optimized and validated. Training and validation sample sets of land cover
type, grassland biomass, leaf area index (LAI), and soil moisture were
established for the intelligent classification and calculation of each feature
covariate. An improved surface water body extraction model based on a
convolutional neural network, an LAI inversion model based on vegetation index
and neural network, a vegetation cover reconstruction model based on random
forest, and a grassland biomass estimation model based on machine learning were
constructed.
In combination
with the screening of international and domestic satellite data sources and the
support of callable resources, the calculation of long time series and
high-resolution key parameter data products were implemented. Collaborative
validation of data products was conducted, and intelligent generation of key
feature parameter products was completed and shared for distribution, allowing
other users or machines to access this data product.

Figure 1 Technical methodological framework of the
dataset development
3 Data Results and Validation
3.1 Data Composition
The
Mongolian Plateau natural resource and environmental scientific dataset
consists of 5 sub-datasets: long-term land cover dataset of the Mongolian
Plateau based on multi-source data and rich sample annotations, an updatable
data revealing changes in land cover types in Mongolia, the data of spring
sandstorm distribution on the Mongolian Plateau (2000?C2021), Mongolia 30 m
resolution grass yield estimation data (2017?C2021), and the data of annual
surface water distribution in the growing season on the Mongolia Plateau from
2013 to 2022. This dataset covers multiple resource and environment-related
data, such as land cover, grassland, dust storms, and water bodies of the
Mongolian Plateau, and can support monitoring long-term ecological and
environmental issues in the region.
3.2 Data Products
For the 13 main categories of the
Mongolian Plateau, forest, shrub, meadow steppe, real steppe, desert steppe,
wetland, water, cropland, built area, bare area, desert, sand, and ice, we developed
a uniform latitude and longitude grid file spanning the Mongolian Plateau,
using remote sensing imagery as a reference, and manually interpreted and
annotated 43,223 samples within each grid cell. In the years 2020, 2015, 2010,
2005, 2000, 1995, and 1990, the numbers of sample points collected were 11,295,
4,521, 4,887, 5,794, 4,459, 9,807, and 2,460, respectively. Among these, 90% of
the sample points were used for model training and 10% for product accuracy
validation. The Google Earth Engine was used as the platform for data
collection and model training. By integrating the sample point data over the
years, images and data from seven periods between 1990 and 2020 were unified. A
feature dataset was constructed??including blue, green, red, near-infrared,
short-wave infrared 1, short-wave infrared 2, NDVI (Normalized Difference Vegetation
Index), NDWI (Normalized Difference Water Index), elevation, slope, and
nighttime light data??to map feature pixel values to label values in a
one-to-one correspondence. A Random Forest algorithm was employed to train the
training point set with 100 trees. The trained Random Forest classifier was then
applied to the prediction image set to produce land cover data products for the
Mongolian Plateau (Figure 2).
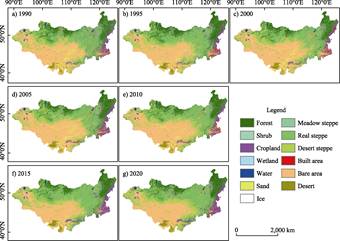
Figure 2 Land cover maps of the
Mongolian Plateau (1990?C2020)
The remote-sensing interpretation was performed
objectively using eCognition. Reference thresholds were set based on the NDVI
for forests, meadow steppe, real steppe, and desert steppe, the normalized
difference soil index for bare areas, the sum of all bands for sand,
compactness for croplands, and NDWI for water bodies. Manual visual inspection
was then performed to adjust the thresholds for identifying deserts, built
areas, and ice[2]. Finally, an 11-category landcover dataset for
Mongolia was constructed (Figure 3).
Using MODIS L1B data, we constructed a normalized
difference dust index, the thermal infrared dust index, the brightness
temperature difference index algorithm, and a threshold-free dust detection
index (DSDI)[3]. The DSDI could effectively extract dust storm
pixels, and for all dust storm events, the DSDI values were greater than zero.
This effectively avoids the problem of threshold differences and makes the
method suitable for the spatial and temporal scales of the Mongolian Plateau.
By applying this index to spring images from 2000 to 2021, we obtained the
spring dust distribution over the Mongolian Plateau for the past 20 years
(Figure 4).

Figure 3 Land cover maps of
Mongolia (1990?C2020)
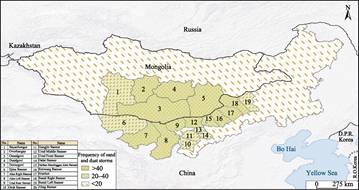
Figure 4 Frequency distribution map
of spring sandstorms in the Mongolian Plateau (2000?C2021)
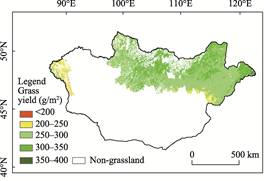
Figure 5 Spatial distribution map of grass yield
in Mongolia (2021)
|
We obtained the necessary remote sensing
images, measured land data, and other relevant information. Combining these
data with the Mongolian Plateau land cover data produced in the previous
section to extract the grassland areas of Mongolia, we used the Google Earth
Engine (GEE) platform for preprocessing to generate the required training
dataset. 4 models were compared: multiple linear regression, random forest,
K-nearest neighbors, and artificial neural networks. The best-performing grass
yield estimation model was selected and applied to grass yield estimation[4]
(Figure 5).
We constructed multichannel synthetic feature
data, including red, green, blue, near- infrared, NDWI, shortwave infrared,
enhanced water index bands, and a digital elevation model. The label noise
correction method was applied to the waterbody information in the quality
assessment band to obtain corrected waterbody labels, which were combined with
the feature data to build a training dataset. A deep learning-based water body
classification model[5] was trained locally. By combining local deep
learning training with GEE distributed computing and parsing the deep learning
model structure and GEE??s function interfaces, we enabled deep learning
capabilities within the GEE[6]. The waterbody classification model
was automatically deployed in GEE for online computation and applied to annual
feature images from 2013 to 2022, completing the acquisition of the annual
growing season surface water distribution of the Mongolian Plateau from 2013 to
2022 (Figure 6).

Figure 6 Temporal and spatial
distributions maps of surface water in the Mongolian Plateau (2017?C2022)
3.3 Data Validation
Mongolian
Plateau land cover: A total of 4,383 validation samples were collected over seven data periods from 1990 to
2020. After the calculations, the overall validation accuracy was 83.9%, and
the Kappa coefficient was 0.817. The average precision was 86.1%, the average
recall was 81.4%, and the weighted F1 score was 84.0%, respectively. Examining
different years, the overall accuracies for 1990, 1995, 2000, 2005, 2010, 2015,
and 2020 were 80.9%, 73.4%, 78.2%, 85.5%, 67.6%, 94.5%, and 97.7%,
respectively. The Kappa coefficients for these
years were 0.78, 0.70, 0.75, 0.83, 0.62, 0.94, and 0.97, respectively.
Mongolian land cover:
The overall classification accuracies of the land cover data for 1990, 2000,
2010, and 2020 were 84.19%, 82.12%, 81.84%, and 81.84%, respectively. The Kappa
coefficients for these years were 0.805,2, 0.765,6, 0.798,5, and 0.799,1,
respectively[2].
Dust storm distribution in the Mongolian
Plateau: After determining the true values of the training sample points, the
accuracy of the dust storm detection index was evaluated using an error matrix.
The overall classification accuracy of the DSDI dust storm detection index for
extracting near-spring dust distribution in the Mongolian Plateau can reach up
to 85.24% with a Kappa coefficient of 0.763,6[7].
Grass
yield of Mongolia: A comparison was made between 4 model methods, i.e.,
artificial neural network (ANN), random forest (RF), K-nearest neighbors (KNN),
and multiple linear regression (MLR). The ANN model exhibited the highest
accuracy (R2= 0.78, root mean square error (RMSE)=48.7 g/m2),
followed by the RF model (R2=0.72, RMSE=55.28 g/m2),
both of which significantly outperformed the other two models. Both models were suitable for estimating grass yield of
Mongolia. The accuracy of the KNN model was slightly lower than these two,
whereas the MLR model could only explain 40% of the variance, which was
slightly better than the statistical models using a single vegetation index.
Therefore, the ANN model was chosen to estimate grass yield of Mongolia[8].
Surface water of the Mongolian Plateau:
Manual screening of validation points was conducted using the Google Earth for
each year from 2013 to 2022, resulting in a total of 5,000 validation samples
over 10 years (limited to 200 water body samples and 300 non-water samples per
year). The total numbers of water bodies and non-water samples were 2,000 and
3,000, respectively. The calculations showed that the overall accuracy was
greater than 86%, with an average Kappa coefficient of 0.75[9].
4 Conclusion
This study focuses on the
Mongolian Plateau, the core area of the China-Mongolia-Russia Economic Corridor
under the ??Belt and Road?? initiative, and proposes a solution centered on Earth
big data technology to address regional ecological and environmental issues.
Resource and environmental scientific data products for the Mongolian Plateau
target different surface parameters by comprehensively utilizing random forest
classifiers, object-oriented interpretation, index threshold classification,
deep learning algorithms, and cloud computing methods. Combined with massive
manually labeled samples and visual discrimination data, we developed multiple
datasets, including land cover, spring dust storm distribution, grass yield
estimation, and surface water distribution. In terms of data accuracy, various
evaluation methods, such as overall accuracy, Kappa coefficient, RMSE, and
confusion matrix, were employed to ensure high precision and reliability of the
data. Specifically, the land cover accuracy for the Mongolian Plateau was
83.9%, Mongolia??s land cover exceeded 80%, the dust storm distribution data had
an accuracy of 85.24%, the RMSE for grass yield was 48.7 g/m2, and
the overall accuracy of the surface water distribution data was better than
86%. The resulting datasets not only provide scientific evidence for ecological
and environmental monitoring of the Mongolian Plateau but also offer important
support for cross-border ecological governance cooperation among China,
Mongolia, and Russia. The outcomes have been applied in the UNESCO Disaster
Risk Reduction Knowledge Service System, as the case report ??Earth Big Data
Supporting the United Nations Sustainable Development Goals?? and the ??100
Excellent Applications of Earth Observation?? by the National Remote Sensing
Center.
Author Contributions
Wang, J. L. created the overall design for the
development of the dataset and drafted the manuscript; Li, K. obtained surface
water data of the Mongolian Plateau and jointly drafted the manuscript;
Altansukh, O. supported the collection of field investigation and validation
data; Xu, S. X. and Wei, H. S. obtained land cover data of the Mongolian
Plateau.
Conflicts of Interest
The
authors declare no conflicts of interest.
References
[1]
Wang, J. L.
Preface of ??resource and environmental data of the Mongolian
Plateau?? [J]. China Scientific Data,
2023, 8(1): 7.
[2]
Wang, J.
L., Wei, H. S., Cheng, K., et al. Updatable dataset revealing decade
changes in land cover types in Mongolia [J]. Geoscience Data Journal,
2022, 9(2): 341?C345. DOI: 10.1002/gdj3.149.
[3]
Zhang, Y.,
Wang, J. L., Altansukh, O., et al. Dynamic evolution of spring sand and
dust storms and cross-border response in Mongolian plateau from 2000 to 2021
[J]. International Journal of Digital Earth, 2023, 16(1): 2341?C2355.
[4]
Li, M. H.,
Wang, J. L., Li, K., et al. Spatial-temporal pattern analysis of
grassland yield in Mongolian Plateau based on artificial neural network [J]. Remote
Sensing, 2023, 15(16): 19. DOI: 10.3390/rs15163968.
[5]
Li, K.,
Wang, J. L., Yao, J. Y. Effectiveness of machine learning methods for water
segmentation with ROI as the label: a case study of the Tuul River in Mongolia
[J]. International Journal of Applied Earth Observation and Geoinformation,
2021, 103(7): 102497. DOI: 10.1016/j.jag.2021.102497.
[6]
Li, K.,
Wang, J. L., Cheng, W. J., et al. Deep learning empowers the Google
Earth Engine for automated water extraction in the Lake Baikal Basin [J]. International
Journal of Applied Earth Observation and Geoinformation, 2022, 112: 102928.
[7]
Zhang, Y.,
Wang, J. L. A dataset of spring sandstorm distribution on the Mongolian Plateau
(2000?C2021) [J/OL]. China Scientific Data, 2023, 8(1): 123?C133. DOI:
10.11922/11-6035.csd.2023.0032.zh.
[8]
Li, M. H., Wang, J. L., Li,
K. A dataset of grass yield
estimation with 30 m resolution in Mongoliaduring 2017?C2021 [J/OL]. China Scientific
Data, 2023, 8(1): 14?C22. DOI:
10.11922/11-6035.csd.2023.0006.zh.
[9]
Li, K.,
Wang, J. L., Cheng, W. J., et al. A
dataset of annual surface water distribution in the growing season on the
Mongolia Plateau from 2013 to 2022 [DS/OL]. Science Data Bank, 2022. DOI: 10.57760/sciencedb.j00001.00665.